DUET Launches Ethical Principles for Using Data-Driven Decision in the Cloud
- Pavel Kogut
- Jan 11, 2023
- 6 min read
Key Points
Local Digital Twins (LDTs) use personal and non-personal data in order to improve urban operations, the environment and economic outcomes within cities.
Despite allowing for increased efficiency and forward city planning, the use of personal data can give rise to exploitation of the rights and interests of individuals, resulting in harm on a personal level.
Therefore, with the support of DUET LDT pilots (Athens, Pilsen, Flanders) who provided a range of findings and real-life data-supported policy case studies, the legal (GSL), management (AIV/DV) and a technical consortium partner (IMEC) drafted a guide on ethical principles for using data-driven decisions in the cloud.
The aim of this guide is to provide future LDTs support in making ethically aware, compliant and legal decisions in regards to data processing.
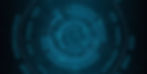
Image courtesy: Pixabay
Abstract
This deliverable seeks to provide the final version of and Ethical Code of Conduct tailored to assist in any data-based decision-making process. DUET LDT pilots undertook interviews with GSL to discuss their usage of the first iteration of the deliverable (D1.5), improvements in the structure and user experience of the guidance, and the evolving nature of use cases. As the second and final iteration, the guidance also includes new user types and the ethical considerations to discuss, including entrepreneur/founder, smart city provider roles, and an extension of a public servant/city official role.
In particular, the guidance discusses the building blocks of the ethical discourse around data-based decision making, and suggests an ethical code of conduct (ethical principles) for LDTs in such a context.
Recommendations
Ethical considerations should be read alongside data protection and privacy aspects. In particular, LDT pilots should be aware of steps to take to ensure a privacy-by design approach, including the data minimisation principle.
Anonymisation or avoidance of personal data is preferable unless it is strictly necessary for your task and proportionate to meeting the pre-defined purpose of your activity. In cases where personal data must be used and anonymisation would make the purpose of the use case futile, the guidance highlights techniques and procedures to be avoided in such a case such as singling out data, aggregated records and personal data by inference.
Consider the interconnected nature of data storage. Despite the focus of DUET on LDTs, use cases should consider the possibility and necessary safeguards if personal data must be stored outside of Europe.
The final thirteen ethical principles can be found below. For the complete context and further guidance for smart cities, reference to the full deliverable is advisable and can be found below.
1. Accountability and data sovereignty
Know the origin of the data, its lawful and ethical uses, and any limitations on their sharing or publication.
This includes understanding the origin of data when working with private/public data sources as expressed by the European Union Agency for Cybersecurity (ENISA).
This also includes understanding all possible locations of processing of personal data and the different data regulations this may be subject to.
2. Transparency
You should know what data you collect and for what purposes.
The data subjects (e.g. the citizens) should know what data you collect about them and for what purposes.
Be transparent about the scope and source of the data, as well as the limitations of the data. Explain what information the data contains, how (and where) it was collected, whether it is static data, updated regularly, or real-time.
If the data is publicly available, provide a link to the origin data repository/source url.
Make sure that decision makers are aware of the deficiencies/limitations of the data.
Promote knowledge of utilisable data/models within your organisation so that employees are aware that helpful data or applications may be available to carry out their tasks.
3. Data quality
Get the best data as you can for your purposes. Best may mean:
data most suited for your purpose;
most complete, correct, and up-to-date data (clean data);
data with a transparent track record of their collection, storage, and the log of previous processing;
data with a clear licence to (further) use.
Take active steps to ensure and maximise the quality, objectivity, usefulness, integrity and security of data.
4. Data quality for publication
If the data is sufficient for an internal use (within the services of the city), it is typically equally good for making the data publicly accessible (open).
Use open standards and open licences.
Publish / share data only after you have cleared the applicable legal requirements.
5. Data security
The integrity and security of data should be maximised.
Use trusted third-party services providers (e.g., approved by the future European Union Cybersecurity Certification Scheme on Cloud Services (EUCS)).
6. Data everywhere
Promote the use of data in public interest, be active in seeking out data that may be (re)used in public interest.
Actively explore the ways in which data can be obtained from partners (private or public) with whom you engaged in a joint activity (e.g., public procurement).
7. Transparent and fair use of AI and computer models. Fighting the “opacity” problem.
Cities should strive to develop the officials’ ability to understand, interpret and use automated decision-making systems. They should understand at least the basics of the underlying algorithms and the data used. This can be achieved by a targeted education and training, for example.
Data subjects (citizens) should be informed about the fact that automated decisions are being taken about them and with the help of their data. To the extent possible, cities should strive to make sure that data subjects also understand the underlying algorithms, to the extent practicable.
Algorithms and automated decisions should be fair and proportional. They should not prejudice the data subjects. Even though some bias may be inherent in data, the algorithms and the data they use (or train on) should not create or perpetuate material biases (racial, ethnical, sexual, political, religious, etc.)
Ensure an element of human control over the AI:
Individuals to whom human oversight is assigned should fully understand the capacities and limitations of the AI system and should be able to duly monitor its operation, so that signs of anomalies, dysfunctions and unexpected performance can be detected and addressed as soon as possible.
Data subjects should be granted the right to appeal relating to data processing and the automated decisions that affect them.
8. Presentation of data or results
The way data or data-based decisions are presented should avoid creating or perpetuating bias (e.g., the use of red and green color coding for visualisations).
9. Data ownership and management
Data ownership typically goes hand in hand with the responsibility for data management.
Third parties contracted out for city data management should be chosen responsibly, adequate data processing agreements should be put in place.
Smart cities should understand if their data is public or private when acquiring a data set from a third party source, and the limitations on usage.
10. Privacy-by-design
Comply with all legal requirements when acquiring, using, or publishing personal data. (see also D1.2 Cities Guide to Legal Compliance for Data-Driven Decision Making).
If you come across a personal data breach, report it to your Data Protection Officer.
Minimise the amount of personal data obtained, used and stored.
11. Anonymised data preference
Do not use personal data unless it is strictly necessary for your task and proportionate to meeting the pre-defined purpose of your activity.
If anonymous data is not available, but personal data is, ensure that the data is anonymised before its further use, if possible. Ask the upstream data provider, who best understands the data, to anonymise the data before it is supplied.
Non-anonymised data should in no case be made public (or open data), unless strictly required for carrying out the task in question, and unless cleared by the Data Protection Officer for publication.
Where data is anonymised, do not proactively take any steps in the direction to re-identify the data (link the data to individual persons). The following techniques and procedures, for example, should be avoided unless the goal is actually to re-identify otherwise anonymous or pseudonymised data:
Singling out, which corresponds to the possibility to isolate some or all records which identify an individual in the dataset;
Linkability, which is the ability to link, at least, two records concerning the same data subject or a group of data subjects (either in the same database or in two different databases). If an attacker can establish (e.g. by means of correlation analysis) that two records are assigned to a same group of individuals but cannot single out individuals in this group, the technique provides resistance against “singling out” but not against linkability; or
Inference, which is the possibility to deduce, with significant probability, the value of an attribute from the values of a set of other attributes.
If the risk of re-identification materialises on a given dataset, take all reasonable steps, seek appropriate expert advice and apply all relevant professional standards in order to mitigate the risk of a privacy breach and further unlawful personal data processing.
12. Training and sufficient data usage information
Ensure to provide sufficient information about the application including how it works and the data the model is sourcing from.
If applicable, provide a contact to the application administrator for possible troubleshooting.
Ensure all people involved have an understanding of these ethical principles.